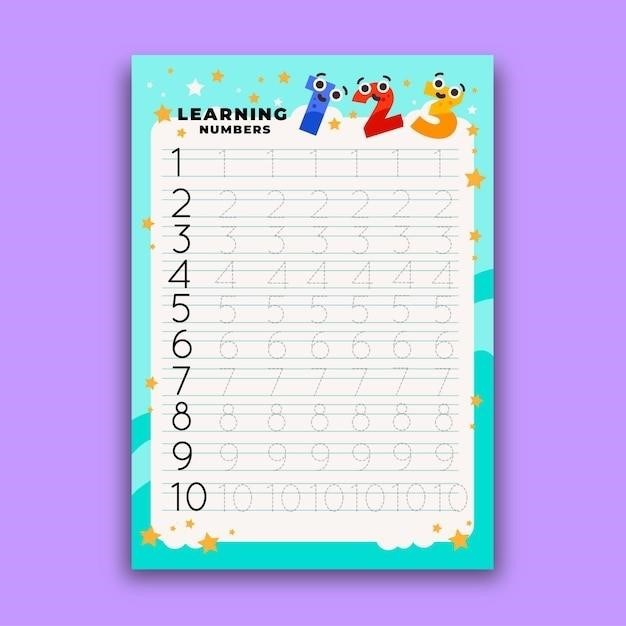
Z-Score Worksheets with Answers⁚ A Comprehensive Guide
Z-scores are a fundamental concept in statistics, providing a standardized way to compare data points within a normal distribution. Understanding z-scores is crucial for analyzing and interpreting data across various fields, including healthcare, finance, and education. This comprehensive guide will cover everything you need to know about z-scores, from their basics to practical applications, including a dedicated section on z-score worksheets with answers to help you solidify your understanding.
Introduction to Z-Scores
In the realm of statistics, z-scores, also known as standard scores, serve as a powerful tool for understanding and comparing data points within a normal distribution. A z-score represents the number of standard deviations a particular data point lies away from the mean of the distribution. Essentially, it quantifies how far a specific observation deviates from the average value, considering the spread of the data. Z-scores are dimensionless, meaning they are independent of the original units of measurement, which allows for meaningful comparisons across different datasets with potentially varying scales.
Imagine a scenario where you have two students, one scoring 85 on a math test and the other scoring 90 on a science test. It’s challenging to directly compare their performances since the tests might have different difficulty levels and scales. This is where z-scores come into play. If we convert their scores into z-scores, we can determine how far each score is from the average performance in their respective subjects, relative to the spread of scores in each subject. A higher z-score indicates a better performance relative to the average, while a lower z-score suggests a performance below the average.
Z-scores provide a standardized framework for data analysis, enabling us to compare and interpret data points across different distributions with ease. They are particularly useful in situations where we need to determine the relative position of a data point within a dataset, or when comparing the performance of individuals or groups across different tests or measurements.
Understanding the Standard Normal Distribution
The foundation of z-scores lies in the concept of the standard normal distribution, often referred to as the bell curve. This distribution is a fundamental concept in statistics, characterized by its symmetrical, bell-shaped curve. It’s a theoretical distribution where the mean, median, and mode coincide, representing the most frequent data point. The standard normal distribution has a mean of 0 and a standard deviation of 1, providing a standardized framework for comparing data points across different datasets.
The area under the standard normal curve represents probabilities. For instance, the area under the curve between two z-scores indicates the probability of obtaining a value within that range. This property is crucial for various statistical applications, such as hypothesis testing, confidence intervals, and determining the likelihood of events. The shape of the standard normal curve is such that approximately 68% of the data falls within one standard deviation of the mean, 95% falls within two standard deviations, and 99.7% falls within three standard deviations. This property is known as the “68-95-99.7 rule” and serves as a useful guideline for understanding the distribution of data.
By transforming raw data into z-scores, we can effectively convert any normal distribution into the standard normal distribution. This allows us to utilize the properties and tables associated with the standard normal distribution to analyze and interpret data from various sources, regardless of their original units of measurement.
Calculating Z-Scores
Calculating z-scores is a straightforward process that involves standardizing a raw score relative to the mean and standard deviation of the distribution. The formula for calculating a z-score is⁚
z = (x ‒ μ) / σ
Where⁚
- z is the z-score
- x is the raw score
- μ is the population mean
- σ is the population standard deviation
The z-score essentially represents how many standard deviations a particular raw score is away from the mean. A positive z-score indicates that the raw score is above the mean, while a negative z-score indicates that the raw score is below the mean.
For example, if a student scores 80 on a test with a mean of 70 and a standard deviation of 10, their z-score would be⁚
z = (80 ‒ 70) / 10 = 1
This means that the student’s score is one standard deviation above the mean.
Calculating z-scores is essential for various statistical applications, including comparing scores from different distributions, identifying outliers, and determining probabilities associated with specific data points.
Interpreting Z-Scores
Z-scores provide a powerful way to interpret data within a normal distribution, offering insights into the relative position of a data point compared to the rest of the distribution. A positive z-score indicates that the data point is above the mean, while a negative z-score indicates it’s below the mean. The magnitude of the z-score reflects the distance from the mean, measured in terms of standard deviations.
For instance, a z-score of 1.5 means the data point is 1.5 standard deviations above the mean, suggesting it falls relatively high within the distribution. Conversely, a z-score of -2.0 implies the data point is 2 standard deviations below the mean, indicating a relatively low position within the distribution.
Interpreting z-scores becomes particularly useful when comparing data points from different distributions with varying means and standard deviations. Z-scores standardize the data, allowing for direct comparisons.
Furthermore, z-scores can be used to determine probabilities associated with specific data points. By referencing a standard normal distribution table or using statistical software, you can find the probability of obtaining a z-score greater than, less than, or within a specific range. This information can be invaluable for hypothesis testing, decision-making, and understanding the likelihood of observing certain outcomes.
In essence, z-scores offer a standardized language for understanding and interpreting data within a normal distribution, providing valuable insights for analysis, comparison, and probability calculations.
Applications of Z-Scores
Z-scores find applications in various fields, proving invaluable for analyzing and interpreting data in diverse contexts. Here are some key applications⁚
Identifying Outliers⁚ Z-scores excel at identifying outliers, data points that deviate significantly from the rest of the distribution. By setting a threshold, typically 2 or 3 standard deviations from the mean, z-scores can flag outliers that might require further investigation. These outliers could represent errors in data collection, unusual events, or potentially valuable insights.
Comparing Data from Different Distributions⁚ Z-scores provide a standardized means to compare data points from different distributions with varying means and standard deviations. This allows for direct comparisons across disparate datasets, enabling more meaningful insights and analysis.
Assessing Performance⁚ Z-scores are widely used in educational and professional settings to assess performance relative to a group. For instance, standardized tests often utilize z-scores to compare individual scores to the average score of the population, providing a standardized measure of relative performance.
Hypothesis Testing⁚ Z-scores play a crucial role in hypothesis testing, allowing researchers to determine the statistical significance of their findings. By calculating z-scores for sample data and comparing them to critical values, researchers can assess whether observed differences between groups are likely due to chance or a true underlying effect.
Quality Control⁚ Z-scores are applied in quality control processes to monitor and analyze product characteristics. By establishing acceptable ranges for z-scores, companies can ensure that products meet quality standards and minimize defects.
In summary, z-scores are a versatile tool with a wide range of applications, enabling data analysis, comparison, outlier detection, performance assessment, hypothesis testing, and quality control across diverse fields.
Z-Score Practice Worksheet
To solidify your understanding of z-scores and gain confidence in their application, practicing with worksheets is highly recommended. Z-score practice worksheets provide a structured way to work through various scenarios involving calculating, interpreting, and applying z-scores. Here’s a breakdown of the typical content you’ll find in such worksheets⁚
Calculating Z-Scores⁚ These exercises involve calculating z-scores for given data points, means, and standard deviations. They test your understanding of the z-score formula and your ability to plug in values accurately.
Interpreting Z-Scores⁚ These questions focus on translating z-scores into meaningful interpretations. You might be asked to determine the probability of a specific z-score occurring, the percentage of data falling above or below a given z-score, or to explain what a particular z-score represents in terms of its distance from the mean.
Applications of Z-Scores⁚ Worksheet problems often present real-world scenarios where z-scores are applied. You may be asked to determine whether a data point is an outlier, compare the performance of individuals or groups using z-scores, or interpret z-scores within the context of quality control or hypothesis testing.
Z-Score Table Usage⁚ Many worksheets include questions that require using a standard normal distribution table (z-table) to find probabilities associated with different z-scores. These exercises reinforce your understanding of how to use the table effectively and how z-scores relate to probabilities.
Word Problems⁚ Z-score practice worksheets frequently incorporate word problems to assess your ability to translate real-world situations into z-score calculations. These problems help you apply your knowledge in a practical context.
By working through a variety of z-score practice worksheets, you’ll strengthen your understanding of this essential statistical concept and become more adept at analyzing and interpreting data across various fields.
Example Problems and Solutions
Let’s dive into some concrete examples of z-score problems with their solutions to illustrate the practical application of this concept. These examples will help you grasp how to calculate, interpret, and utilize z-scores in various situations.
Example 1⁚ A group of students took a standardized test with a mean score of 75 and a standard deviation of 10. One student scored an 82 on the test. What is the student’s z-score, and what does it tell us about their performance relative to the group?
Solution⁚ The z-score formula is⁚ z = (x ౼ μ) / σ. Plugging in the values, we get⁚ z = (82 ‒ 75) / 10 = 0.7. A z-score of 0.7 means the student’s score is 0.7 standard deviations above the mean. This suggests the student performed slightly better than the average student on the test.
Example 2⁚ A company produces light bulbs with a mean lifespan of 1000 hours and a standard deviation of 100 hours. What percentage of light bulbs have a lifespan exceeding 1200 hours?
Solution⁚ First, calculate the z-score for a lifespan of 1200 hours⁚ z = (1200 ‒ 1000) / 100 = 2. This means a lifespan of 1200 hours is 2 standard deviations above the mean. Using a standard normal distribution table, we find that approximately 2.28% of data points fall above a z-score of 2. Therefore, about 2.28% of the light bulbs produced by the company have a lifespan exceeding 1200 hours.
These examples demonstrate how z-scores can be used to compare individual data points to the overall distribution, assess the probability of certain values occurring, and make meaningful interpretations within a specific context.
Z-Score Table Usage
A crucial tool for working with z-scores is the standard normal distribution table, also known as the z-table. This table provides the area under the standard normal curve, representing the probability of obtaining a z-score within a specific range. Understanding how to read and utilize this table is essential for accurately interpreting z-scores and solving related problems.
The z-table is organized with rows representing the z-score’s whole number and first decimal place, and columns representing the second decimal place. For example, to find the area under the curve corresponding to a z-score of 1.23, you would locate the row for 1.2 and the column for 0.03. The intersection of this row and column would contain the desired area, which represents the probability of obtaining a z-score less than 1.23.
The z-table can also be used to determine the probability of obtaining a z-score greater than a given value. Since the total area under the standard normal curve is 1, you can subtract the area obtained from the table from 1 to get the probability of a z-score exceeding the specified value.
For instance, if you need to find the probability of a z-score greater than 1.23, you would first obtain the area corresponding to a z-score of 1.23 from the table. Subtracting this area from 1 will give you the probability of a z-score exceeding 1.23. Mastering the use of the z-table is fundamental to efficiently and accurately working with z-scores and applying them in various statistical scenarios.
Common Z-Score Scenarios
Z-scores are versatile tools that find applications in various scenarios within statistics and data analysis. Let’s explore some common situations where z-scores prove invaluable⁚
One frequent scenario involves comparing individual data points to the mean of a distribution. For example, if you know the average height of adult males in a particular country and the standard deviation, you can calculate the z-score for a specific individual’s height. This z-score reveals how many standard deviations the individual’s height is above or below the average, allowing for a standardized comparison across different populations.
Another common use case involves determining the probability of observing a particular value within a distribution. For example, if you are given a z-score and need to find the probability of obtaining a value greater than or less than that z-score, you can use the standard normal distribution table to find the corresponding area under the curve. This information can be valuable in quality control, where you might want to determine the probability of a manufactured product exceeding a specific quality threshold.
Furthermore, z-scores are crucial in hypothesis testing, where they are used to assess the statistical significance of results. By comparing a calculated z-score to a critical z-score, you can determine if the observed difference between groups or samples is statistically significant or likely due to chance.
These are just a few examples of the many common scenarios where z-scores play a vital role. Understanding their applications and how to calculate and interpret them is essential for anyone working with data and making informed decisions based on statistical analysis.
Real-World Applications of Z-Scores
Z-scores extend beyond theoretical calculations and find practical applications in various real-world scenarios, impacting our daily lives and influencing crucial decisions across numerous fields. Let’s delve into some compelling examples⁚
In healthcare, z-scores play a critical role in monitoring patient health and identifying potential issues. For instance, pediatricians use z-scores to assess a child’s growth and development, comparing their height, weight, and head circumference to age-specific norms. A child with a significantly low or high z-score might require further investigation or intervention to ensure their well-being.
The financial industry utilizes z-scores to assess risk and manage investments. For example, credit scoring models often incorporate z-scores to evaluate the creditworthiness of individuals or businesses. A higher z-score might indicate a lower risk of defaulting on loans, while a lower z-score might signal a higher risk. This information helps lenders make informed decisions about loan approvals and interest rates.
Z-scores are also employed in quality control to monitor production processes and ensure the consistency of manufactured products. By calculating the z-scores for key quality metrics, manufacturers can identify deviations from desired standards and implement corrective actions to improve quality and minimize defects.
Beyond these examples, z-scores find applications in fields like education, where they are used to evaluate students’ performance relative to their peers, and in sports analytics, where they help quantify athletes’ performance and identify areas for improvement. The versatility of z-scores makes them a valuable tool for analyzing data and making informed decisions across diverse domains.